Learner corpus research: a fast-growing interdisciplinary field
Sylviane Granger
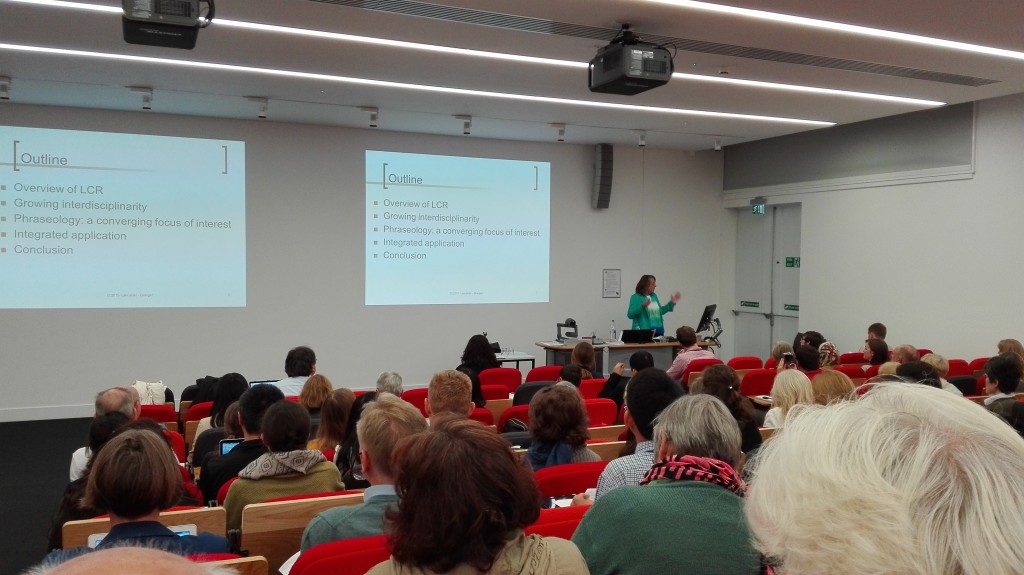
LCR IS an interdisciplinary research
Design: learner and taks variables to control
Not only English language
Method: CIA (Granger, 1996) and computer-aided error analysis
Wider spectrum of linguistic analysis
Interpretation: focus on transfer but this is changing; growing integration of SLA theory
Applications: few up-and-running resources but great potential
Version 3 (2016 or 2017) around 30 L1s as opposed to 11 L1s in Version 1
Learner corpora is a powerful heuristic resource
Corpus techniques make it possible to uncover new dimensions of learner language and lead to the formulation of new research questions: the L2 phrasicon (word combinations).
Prof. Granger brings up Leech’s preface to Learner English on Computer (1998)
Gradual change from mute corpora to sound aligned corpora
POS tagging has improved so much
Error-tagging: wide range of error tagging systems: multi-layer annotation systems
Parsing of learner data (90% accuracy Geertzen et al. 2014)
Static learner corpora vs monito corpora
CMC learner corpus (Marchand 2015)
Granger (2009) paper on the learner research field:
Granger, Sylviane. “The contribution of learner corpora to second language acquisition and foreign language teaching.” Corpora and language teaching 33 (2009): 13.
CIA V2 Granger (2015): a new model
SLA researchers are more interested in corpus data and corpus linguists are more familiar with SLA grounding
Implications are much more numerous than applications
Links with NLP: spell and gramar checking, learner feedback, native language id, etc.
Multiple perspectives on the same resource: richer insights and more powerful tools
Phraseology
Louvain English for Academic Purposes Dictionary (LEAD)
web-based
corpus based
descriptions of cross-disciplinary academic vocabulary
1200 lexical times around 18 functions (contrast, illustrate, quote, refer, etc.)
A really exciting application